Bienvenue à EGC 2012
|
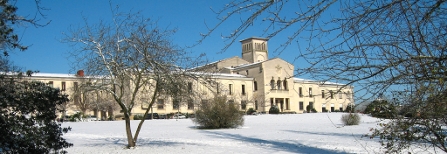 |
12e Conférence Internationale Francophone sur l'Extraction et la Gestion des Connaissances
31 janvier - 3 février, Bordeaux, France
Présidente d'honneur
Wei Wang, University of North Carolina
Mining Genetic Interactions in Genome-Wide Association Study
Résumé :
Advanced biotechnologies have rendered feasible high-throughput data collecting in human and other model organisms. The availability of such data holds promise for dissecting complex biological processes. Making sense of the flood of biological data poses great statistical and computational challenges.
I will discuss the problem of mining gene-gene interactions in high-throughput genetic data. Finding genetic interactions is an important biological problem since many common diseases are caused by joint effects of genes. Previously, it was considered intractable to find genetic interactions in the whole-genome scale due to the enormous search space. The problem was commonly addressed using heuristics which do not guarantee the optimality of the solution. I will show that by utilizing the upper bound of the test statistic and effectively indexing the data, we can dramatically prune the search space and reduce computational burden. Moreover, our algorithms guarantee to find the optimal solution. In addition to handling specific statistical tests, our algorithms can be applied to a wide range of study types by utilizing convexity, a common property of many commonly used statistics.
Biographie:
Wei Wang is a professor in the Department of Computer Science and a member of the Carolina Center for Genomic Sciences at the University of North Carolina at Chapel Hill. She received a MS degree from the State University of New York at Binghamton in 1995 and a PhD degree in Computer Science from the University of California at Los Angeles in 1999. She was a research staff member at the IBM T. J. Watson Research Center between 1999 and 2002. Dr. Wang's research interests include data mining, bioinformatics, and databases. She has filed seven patents, and has published one monograph and more than one hundred research papers in international journals and major peer-reviewed conference proceedings. Dr. Wang received the IBM Invention Achievement Awards in 2000 and 2001. She was the recipient of a UNC Junior Faculty Development Award in 2003 and an NSF Faculty Early Career Development (CAREER) Award in 2005. She was named a Microsoft Research New Faculty Fellow in 2005. She was recently honored with the 2007 Phillip and Ruth Hettleman Prize for Artistic and Scholarly Achievement at UNC. Dr. Wang is an associate editor of the IEEE Transactions on Knowledge and Data Engineering and ACM Transactions on Knowledge Discovery in Data, and an editorial board member of the International Journal of Data Mining and Bioinformatics. She serves on the program committees of prestigious international conferences such as ACM SIGMOD, ACM SIGKDD, VLDB, ICDE, EDBT, ACM CIKM, IEEE ICDM, and SSDBM.
Publications and home page of Wei Wang : http://www.cs.unc.edu/~weiwang/
Conférenciers invités
Daniel Keim, University of Konstanz
Solving Problems with Visual Analytics: Challenges and Applications
Résumé :
Never before in history data is generated and collected at such high volumes as it is today.As the volumes of data available to business people, scientists, and the public increase,their effective use becomes more challenging. Keeping up to date with the flood of data,using standard tools for data analysis and exploration, is fraught with difficulty. The field ofvisual analytics seeks to provide people with better and more effective ways to understandand analyze large datasets, while also enabling them to act upon their findings immediately. Visual analytics integrates the analytic capabilities of the computer and the abilities of the human analyst, allowing novel discoveries and empowering individuals to take control of the analytical process. Visual analytics enables unexpected and hidden insights, which may lead to beneficial and profitable innovation. The talk presents the challenges of visual analytics and exemplifies them with application examples, illustrating the exiting potential of current visual analysis techniques.
Biographie:
Daniel A. Keim is full professor (Chair of Information Processing) at the Computer Science department of the University of Konstanz. After being assistant professor at the University of Munich, and later at the Martin- Luther-University Halle, he was senior researcher at AT&T Shannon Research Labs, USA. Professor Keim's publication have had impact in data mining, information visualization and visual analytics. He is an editor of TKDE and the Information Visualization Journal and is part of the IEEE InfoVis, IEEE VAST and IEEE/EG EuroVis annual conferences steering committees.
Publications and home page of Daniel Keim :
http://www.informatik.uni-konstanz.de/arbeitsgruppen/infovis/mitglieder/prof-dr-daniel-keim/
Donato Malerba, University of Bari
Relational Learning from Spatial Data: Retrospect and Prospect
Résumé :
Learning from spatial data is characterized by two main features.
First, spatial objects have a locational property which implicitly
defines several spatial relationships (topological, directional, distancebased)
between objects. Second, attributes of spatially related units tend
to be statistically correlated. These two features argue against the assumption
of the independent generation of data samples (i.i.d. assumption)
underlying classic machine learning algorithms, and motivate the
application of relational learning algorithms, whose inferences are based
on both instance properties and relations between data. This relational
learning approach to spatial domains has already been investigated in
the last decade, and important accomplishments in this direction have
already been performed. In this talk, we retrospectively survey major
achievements on relational learning from spatial data and we report open
problems which still challenges researchers and prospectively suggest important
topics for incorporation into a research agenda.
Biographie:
Donato Malerba is a full professor at the Department of Informatics, University of Bari, where he teaches in the courses of "Algorithms and Data Structures", "Advanced Data Base Systems", and "Knowledge Bases and Data Mining". In 1992 he was assistant specialist at the Institute of Computer Science, University of California, Irvine. His research activity mainly concerns machine learning and data mining, in particular numeric-symbolic methods for inductive inference, classification and model trees, (multi-)relational data mining, spatial data mining, web mining, and their applications to intelligent document processing and digital map interpretation. He has published more than 150 papers in international journals and conference proceedings.
He was in the Management Board of the European Coordinated Action FP6-021321 "KDUbiq - Knowledge Discovery in Ubiquitous Environments" (December 2005 - May 2008) and in the Management Board of the European project IST-2001-33086 "KDNet - European Knowledge Discovery Network of Excellence" (2002 - 2004). He participated to several European and National projects. He was responsible of the unit of Bari in the European project IST-1999-10536 SPIN (Spatial Mining on Data of Public Interest) and in two MIUR COFIN projects (years 1999-2001, 2001-2003).
He is responsible of a research unit of the strategic project PS121 "Telecommunication Facilities and Wireless Sensor Networks in Emergency Management" funded by Apulia Region.
He has received the IBM Faculty Award for the year 2004.
He has been in the executive board of the Italian Association for Artificial Intelligence (AI*IA) from September 2001 till September 2005.
He has served in the program committee of many international conferences and workshops of machine learning and data mining, co-chaired seven international/national workshops and acted as guest-editor of six special issues of international journals (topics: machine learning in computer vision, mining official data, visual data mining, spatio-temporal data mining, artificial intelligence, multi-relational data mining).
He was program co-chair of the 18th International Conference on Industrial & Engineering Applications of Artificial Intelligence and Expert Systems (IEA-AIE'05), Bari, June 2005, and of the 16th International Symposium on Methodologies for Intelligent Systems (ISMIS'06), Bari, September 2006. He is program co-chair of the conference ECML/PKDD which will be held in Athens, Greece, next September 2011. He is in the editorial board of Machine Learning Journal, Journal of Intelligent Information Systems, and International Journal of Data Mining, Modelling and Management.
Publications and home page of Donato Malerba : http://www.di.uniba.it/~malerba/
Helen Purchase, University of Glasgow
User Evaluation: Why?
Résumé :
Research in information visualisation has changed significantly in the past two
decades. Once it was sufficient to simply design and implement an impressive
visualisation system. Today editors and reviewers expect papers to present not
only a novel system, but empirical evidence of its worth. Why has this change
come about, and what impact has it had on those working in this area? This
talk will discuss how a field dominated by algorithms and tools became infected
by human participants, and why this is a positive development in a maturing
research discipline.
Biographie:
Dr Helen Purchase is Senior Lecturer in the School of Computing Science at the University of Glasgow. She has worked in the area of empirical studies of graph layout for several years, and also has research interests in visual aesthetics, task-based empirical design, collaborative learning in higher education, and sketch tools for design. She is currently writing a book on empirical methods for HCI research.
Publications and home page of Helen Purchase : http://www.dcs.gla.ac.uk/~hcp/
Michel Tenenhaus, HEC Paris
PLS path modeling and regularized generalized canonical correlation analysis for multi-block data analysis
Résumé :
Regularized generalized canonical correlation analysis (RGCCA) is a generalization of regularized canonical correlation analysis to three or more sets of variables. It constitutes a general framework for many multi-block data analysis methods. It combines the power of multi-block data analysis methods (maximization of well identified criteria) and the flexibility of PLS path modeling (the researcher decides which blocks are connected and which are not). Searching for a fixed point of the stationary equations related to RGCCA, a new monotone convergent algorithm, very similar to the PLS algorithm proposed by Herman Wold, is obtained. Finally, a practical example is discussed.
Biographie:
Michel Tenenhaus is Professor emeritus of Statistics at HEC Paris. His main researches are concerned with multivariate data analysis: optimal scaling methods for categorical variables, PLS regression, PLS path modelling and regularized generalized canonical correlation analysis. He has published many papers in scientific journals and three books: Méthodes Statistiques en Gestion (Dunod, 1994), La régression PLS : théorie et applications (Technip, 1998) and Statistique: Méthodes pour décrire, expliquer et prévoir (Dunod, 2007). Michel Tenenhaus is also consultant for industrial companies. He has been chairman of PLS'99 at Jouy-en-Josas and PLS'09 in Beijing, and co-chairman of the following symposia PLS'01 at Anacapri, PLS'03 at Lisbon, and PLS'05 at Barcelona.
Publications and home page of Michel Tenenhaus : http://www.hec.edu/Faculty-and-Research/Faculty/TENENHAUS
Tutoriels invités
Antoine Cornuéjols, AgroParisTech
Apprentissage artificiel : mise en perspective d'un demi-siècle d'évolution
Résumé :
...
Biographie:
Antoine Cornuéjols est Professeur en Informatique à AgroParisTech dans le département "Modélisation Mathématiques, Informatique et Physique". Après un Master of Sciences à UCLA, il a obtenu son doctorat à l'Université d'Orsay. Ses centres d'intérêt incluent l'apprentissage en-ligne et le transfert, les processus de raisonnement en sciences cognitives, la bio-informatique et l'étude du phénomène de transition de phase en apprentissage. Il a donné de nombreux séminaires et conférences invitées sur ces sujets. Il est co-auteur, avec Laurent Miclet, d'un ouvrage de référence "Apprentissage artificiel. Concepts et algorithmes" (Eyrolles, 2ème ed. 2010), ainsi que co-auteur avec Lorenza Saitta et Attilio Giordana de "Phase Transitions in Machine Learning", (Cambridge University Press, 2011).
Publications et page personnelle de Antoine Cornuéjols : http://www.lri.fr/~antoine/
Helen Purchase, University of Glasgow
User Evaluation: How?
Résumé :
...